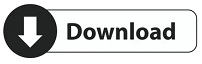
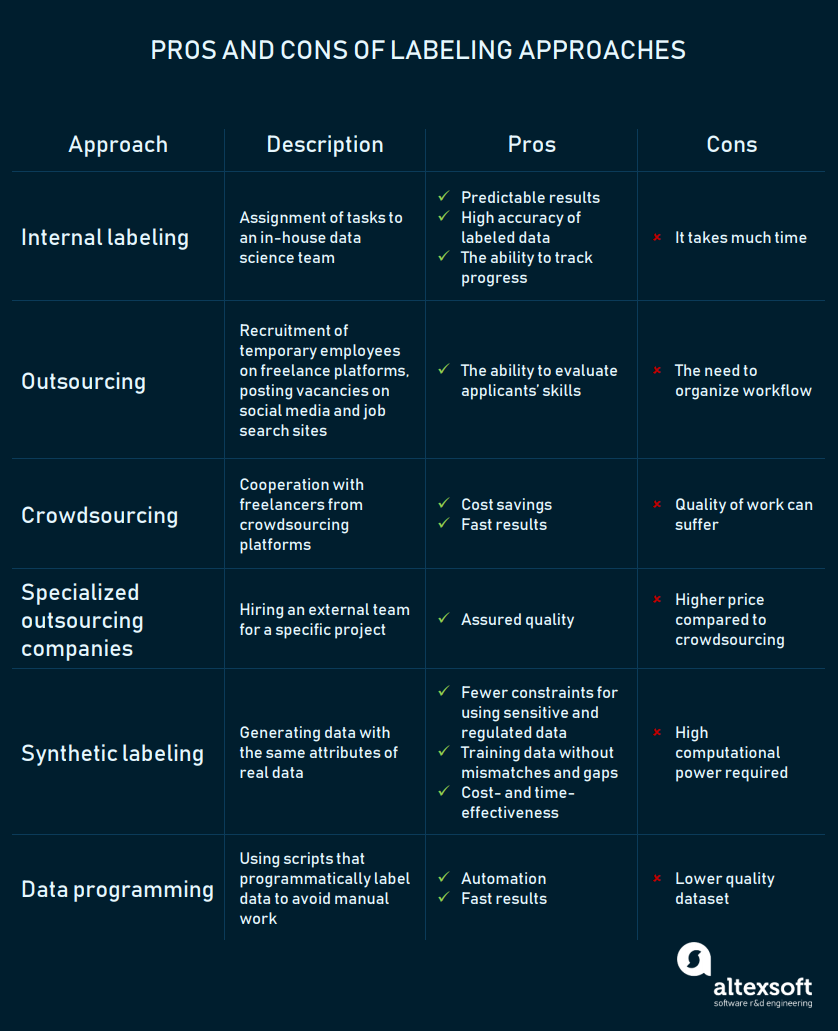
#Data labelling and annotation pro
💡 Pro tip: Check out 21+ Best Healthcare Datasets for Computer Vision if you are looking for medical data.Īs is expected for in-house labeling, with the increase in quality of the annotations, the time taken to annotate increases drastically, resulting in the entire data labeling process and cleaning being very slow. High-quality labeling is crucial for industries like insurance or healthcare, and it often requires consultations with experts in corresponding fields for proper labeling of data. In-house data labeling secures the highest quality labeling possible and is generally done by data scientists and data engineers hired at the organization. The most common approaches for annotation of data are listed below. While internal labeling and crowdsourcing are very common, the terminology can also extend to include novel forms of labeling and annotation that make use of AI and active learning for the task. depending on the problem statement, the time frame of the project, and the number of people who are associated with the work. At times model performance and predictions are validated by a human and the results of the validation are fed back to the model.
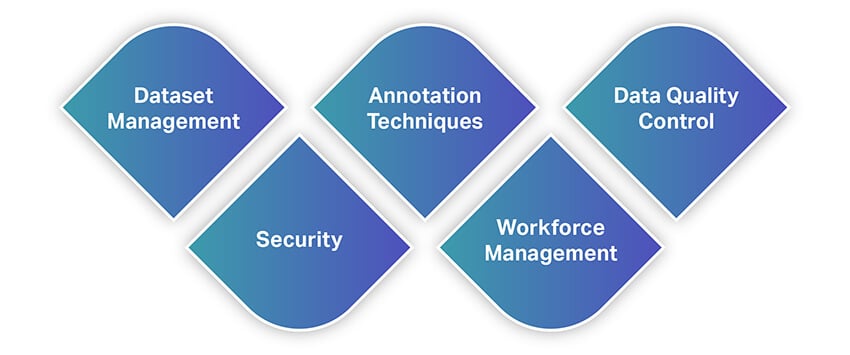
Unsupervised Learning: What’s the Difference? What is ‘Human-in-the-Loop’ (HITL)? 💡 Pro tip: Dive deeper and check out Supervised vs. Use cases of semi-supervised learning include Protein sequence classification and Internet content analysis. While this reduces the cost of data annotation by using both kinds of data, there are generally a lot of severe assumptions of the training data made while training. In semi-supervised learning, a combination of both annotated and unannotated data is used for training the model. Unsupervised learning methods also include clustering algorithms that groups the data into ‘n’ clusters, where ‘n’ is a hyperparameter. In unsupervised learning, unannotated input data is provided and the model trains without any knowledge of the labels that the input data might have.Ĭommon unsupervised algorithms of training include autoencoders that have the outputs the same as the input. Thus, annotated data is an absolute necessity for training machine learning models in a supervised manner. To find the accuracy of such a method, annotated data with hidden labels is typically used in the testing stage of the algorithm. The typical training procedure consists of feeding annotated data to the machine to help the model learn, and testing the learned model on unannotated data. Popular tasks like image classification and image segmentation come under this paradigm. Supervised learning, the most common type, is a type of machine learning algorithm that requires data and corresponding annotated labels to train. Machine/ Deep Learning algorithms can be broadly classified on the type of data they require in three classes. The training dataset is completely dependent on the type of machine learning task we want to focus on. “Ground truth” as a term is used for information that is known beforehand to be true. 💡 Pro tip: Are you looking for quality datasets to label and train your models? Check out the list of 65+ datasets for machine learning. When training data is annotated, the corresponding label is referred to as ground truth. Training data can be of various forms, including images, voice, text, or features depending on the machine learning model being used and the task at hand to be solved. Training data refers to data that has been collected to be fed to a machine learning model to help the model learn more about the data. What is “training data” in machine learning? These tags form a representation of what class of objects the data belongs to and helps a machine learning model learn to identify that particular class of objects when encountered in data without a tag.

Ready to streamline AI product deployment right away? Check out:ĭata labeling refers to the process of adding tags or labels to raw data such as images, videos, text, and audio.
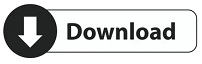